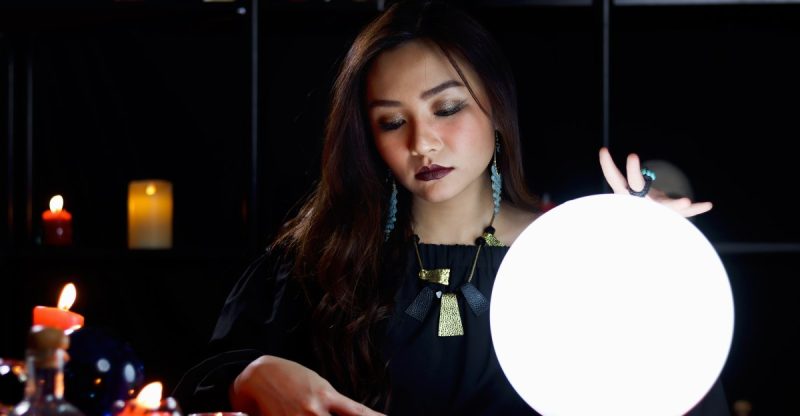
Predicting the future is a human endeavor that has fascinated us for centuries. From predicting stock market peaks to anticipating geopolitical events, the quest for foresight is ongoing. While some individuals, known as superforecasters, demonstrate exceptional predictive abilities, even they are occasionally surprised by unforeseen circumstances. The rise of artificial intelligence (AI) has introduced a new player in the forecasting game, raising the question: can AI outperform humans in predicting the future?
Recent years have witnessed an increasing reliance on AI-powered prediction bots. Financial markets have long utilized algorithmic trading, but the application of AI as a more general forecasting tool is relatively new. However, current evidence suggests that top human forecasters still maintain a competitive edge over their AI counterparts. Competitions like those held by Metaculus, a leading prediction website, consistently show human superforecasters outperforming even the most sophisticated AI bots.
The gap, however, is narrowing. Interestingly, one of the most successful AI models in a recent Metaculus competition employed a surprisingly simple approach: it combined news articles with the predictive capabilities of a large language model (LLM). While this method didn’t surpass human forecasters, it demonstrated significant potential and outperformed many more complex AI models. This highlights the rapid pace of AI development and the potential for future improvements.
The core of AI forecasting lies in machine learning models that analyze vast datasets to predict future events. Generative models, such as those used in chatbots, predict the next word or image, while others predict financial market trends. Many current AI forecasting efforts leverage the power of large language models developed by companies like OpenAI and Google DeepMind, taking advantage of their extensive pre-training rather than starting from scratch. This highlights a key advantage: access to massive datasets and computational resources.
Despite these advancements, significant challenges remain. One major limitation is the inherent difficulty in imbuing AI models with robust quantitative reasoning and logical abilities. For example, the ability to maintain internal consistency across predictions, a crucial aspect of forecasting, is not yet fully realized in AI. However, emerging reasoning models are showing promise in addressing this limitation.
Another significant challenge is the AI’s current limitations in web research. Even advanced models struggle with basic research tasks, such as extracting specific data points or locating datasets. This highlights the crucial role of efficient information gathering in accurate forecasting, a skill in which humans still hold a significant advantage. While AI is rapidly improving, the quality of web research is currently a significant bottleneck limiting its forecasting capabilities.
Despite these limitations, the progress is undeniable. AI models are already approaching human-level forecasting accuracy, and some experts believe that by combining advanced AI with proven forecasting techniques, it may soon surpass human capabilities. This is partly because humans are inherently flawed forecasters, prone to biases and cognitive shortcuts that impede accurate prediction. AI’s potential to overcome these limitations is significant.
The question of whether the world truly needs AI superforecasters remains open. While human superforecasters haven’t yet revolutionized decision-making in areas like government or finance, the potential of AI is different. The speed and scalability of AI could transform how we approach forecasting, offering near-instantaneous predictions for a wide range of scenarios. Imagine a doctor using AI to instantly assess a patient’s prognosis, or a company using it to assess political risk in international operations. The applications are vast.
However, widespread adoption hinges on trust and transparency. The “black box” nature of many LLMs presents a challenge, as their decision-making processes are often opaque. Building trust in AI predictions will require not only demonstrably superior accuracy but also clear and understandable explanations of the models’ reasoning. This is a crucial step towards integrating AI forecasting into mainstream decision-making.
The future of forecasting is likely to be a collaborative one, where human expertise and AI capabilities complement each other. AI’s speed and efficiency could augment human judgment, leading to more accurate and timely predictions. As AI continues to evolve, its role in shaping our understanding of the future will undoubtedly grow, raising both exciting possibilities and important ethical considerations.